MPI-SWS alumni in leadership positions
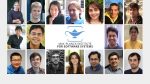
Since its founding at the end of 2004, MPI-SWS has been fortunate to have been the academic home of many amazing doctoral students and postdoctoral fellows. With the ten-year anniversary of the graduation of our first students coming up soon, we wanted to take a moment to celebrate some of the achievements of our alumni:
- Alan Mislove (PhD 2009) is now an Associate Professor at Northeastern University in Boston, Massachusetts,
Since its founding at the end of 2004, MPI-SWS has been fortunate to have been the academic home of many amazing doctoral students and postdoctoral fellows. With the ten-year anniversary of the graduation of our first students coming up soon, we wanted to take a moment to celebrate some of the achievements of our alumni:
- Alan Mislove (PhD 2009) is now an Associate Professor at Northeastern University in Boston, Massachusetts, USA.
- Andreas Haeberlen (PhD 2009) is now an Associate Professor at the University of Pennsylvania in Philadelphia, Pennsylvania, USA.
- Bryan Ford (Postdoc 2009) is now an Associate Professor at EPFL in Lausanne, Switzerland.
- Meeyoung Cha (Postdoc 2010) is now an Associate Professor at KAIST in Daejon, South Korea.
- Boris Köpf (Postdoc 2010) is now a researcher at Microsoft Research Cambridge in the UK.
- Matthew Hammer (PhD 2012) is now an Assistant Professor at The University of Chicago in Illinois, USA.
- Chung-Kil Hur (Postdoc 2012) is now an Associate Professor at Seoul National University in Seoul, South Korea.
- Neel Krishnaswami (Postdoc 2012) is now a Lecturer at the University of Cambridge in the UK.
- Nuno Santos (PhD 2013) is an Assistant Professor at IST, University of Lisbon in Portugal.
- Beta Ziliani (PhD 2013) is a faculty member at Universidad Nacional de Córdoba and a researcher at CONICET in Argentina.
- Aaron Turon (Postdoc 2014) heads the the Rust Development Team at Mozilla Research. Aaron is based in Portland, Oregon, USA.
- Pedro Fonseca (PhD 2015) is an Assistant Professor at Purdue University in West Lafayette, Indiana, USA.
- Pramod Bhatotia (PhD 2015) is a Senior Lecturer at the University of Edinburgh in Scotland, UK.
- Cheng Li (PhD 2016) is a faculty member at the USTC (The University of Science and Technology of China) in Hefei, China.
- Dmitry Chistikov (Postdoc 2016) is an Assistant Professor at the University of Warwick in the UK.
- Rayna Dimitrova (Postdoc 2017) is an Assistant Professor at the University of Leicester in the UK.
- Sadegh Soudjani (Postdoc 2017) is an Assistant Professor at Newcastle University in the UK.
- Vinayak Prabhu (Postdoc 2017) is an Assistant Professor at Colorado State University in Fort Collins, Colorado, USA.
- Mainack Mondal (PhD 2017) is a faculty member at IIT Kharaghpur in West Bengal, India.
- Oana Goga (Postdoc 2017) is a researcher at CNRS in Grenoble, France.
- Riju Sen (Postdoc 2017) is an Assistant Professor at IIT Delhi in India.
- Ori Lahav (Postdoc 2017) is a Senior Lecturer at Tel Aviv University in Israel.
- Mitra Nasri (Postdoc 2018) is an Assistant Professor at the Delft University of Technology in the Netherlands.
Congratulations, everyone! We are proud that MPI-SWS alumni have spread far and wide, pursuing successful research careers all across the globe.